Machine Learning Latest Updates: Innovations and Trends in 2023
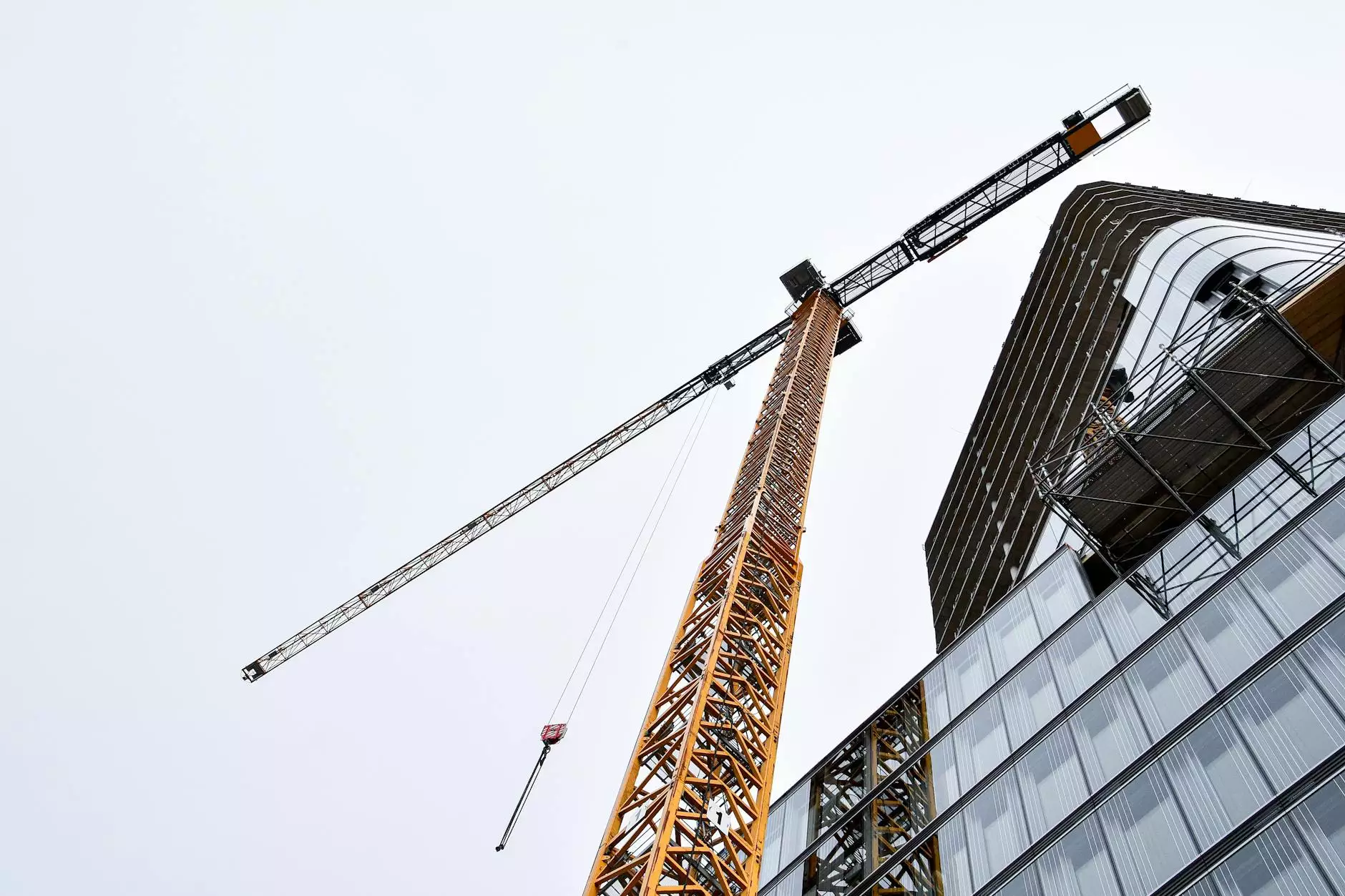
Machine learning is a rapidly evolving field that has significant implications for businesses, technology, and the global economy. In this article, we will delve into the latest updates in machine learning and explore how they are shaping industries and driving innovation in 2023.
The Evolution of Machine Learning
The journey of machine learning began decades ago, but it has gained unprecedented momentum in the last few years. As businesses look to harness the power of data, machine learning has emerged as a fundamental tool. The latest updates illustrate a shift towards more sophisticated algorithms, better data handling, and the integration of machine learning with other advanced technologies.
Key Milestones in Machine Learning Development
- Deep Learning Expansion: Over the past few years, deep learning has made significant strides. The latest frameworks and architectures allow for more efficient training of neural networks, leading to breakthroughs in areas like natural language processing and computer vision.
- AI Ethics and Governance: With great power comes great responsibility. The latest updates show an increased focus on ethical considerations surrounding AI and machine learning, with guidelines and frameworks being established to ensure responsible deployment.
- Transfer Learning Techniques: Transfer learning has gained popularity as it allows models trained on one task to be adapted for another. This not only reduces the amount of data needed but also shortens training times significantly.
Emerging Trends in Machine Learning for Businesses
Businesses across industries are recognizing the importance of leveraging machine learning for competitive advantage. Here are some of the most significant trends observed in 2023:
1. Automated Machine Learning (AutoML)
AutoML tools are becoming increasingly popular, allowing businesses to automate the process of applying machine learning to real-world problems. By democratizing access to machine learning, even those without a data science background can implement effective solutions.
2. AI and Machine Learning in Cybersecurity
As cyber threats become more sophisticated, businesses are turning to machine learning for enhanced security measures. Machine learning algorithms can help identify anomalies and potential threats in real-time, providing businesses with the tools they need to safeguard their data.
3. Personalization through Machine Learning
Consumers increasingly expect personalized experiences, and machine learning is at the forefront of delivering these. Businesses are utilizing machine learning to analyze user behavior and preferences, providing tailored recommendations and services that enhance customer satisfaction.
4. Natural Language Processing (NLP) Advancements
Recent updates in NLP have allowed businesses to leverage chatbots and virtual assistants more effectively. Improved sentiment analysis and understanding of context have made these tools invaluable for customer service, sales, and marketing.
Incorporating Machine Learning in Business Strategy
Successful integration of machine learning into business practices demands a strategic approach. Here are essential steps organizations should consider:
1. Define Clear Objectives
Before diving into machine learning projects, businesses must define what they hope to achieve. Whether it’s improving operational efficiency, enhancing customer experience, or developing new products, clear objectives guide the machine learning initiatives.
2. Invest in Quality Data
Data is the lifeblood of machine learning. Businesses must invest in collecting high-quality, relevant data. This includes not only customer data but also operational data that can provide insights into internal processes.
3. Foster Collaboration Between Departments
A successful machine learning initiative requires collaboration between IT, data science, and business units. Breaking down silos ensures that insights gained from machine learning can be effectively utilized throughout the organization.
4. Continuous Learning and Iteration
Machine learning is not a set-it-and-forget-it solution. Businesses must commit to continuous learning and iteration based on performance data. This allows organizations to refine their models and maintain alignment with changing business needs and market conditions.
Real-World Applications of Machine Learning
To illustrate the tangible benefits of machine learning, here are some examples of companies leveraging the latest updates to their advantage:
1. Healthcare Innovations
In the healthcare sector, machine learning is revolutionizing patient care. From predictive analytics that foresee patient admissions to machine learning algorithms that assist in diagnosing diseases, the potential is immense. For instance, companies are using machine learning to analyze medical images, helping radiologists detect conditions like cancer earlier and more accurately.
2. Financial Services
The financial sector is utilizing machine learning for a variety of tasks, from fraud detection to risk assessment. Algorithms analyze transactions in real-time, identifying suspicious behavior patterns and safeguarding customer assets. Additionally, machine learning helps in personalizing banking services, offering tailored financial products to customers based on their spending habits and credit history.
3. Retail and E-commerce
Retailers have adopted machine learning to enhance inventory management and create personalized shopping experiences. Recommendation engines analyze user interactions and preferences, providing tailored suggestions that drive sales. In addition, predictive analytics help retailers manage their supply chain effectively, minimizing waste and optimizing stock levels.
Machine Learning Challenges and How to Overcome Them
Despite the enormous potential of machine learning, several challenges hinder its effective implementation:
1. Data Privacy Concerns
As machine learning relies heavily on data, privacy concerns are paramount. Companies must ensure compliance with regulations like GDPR and CCPA while protecting customer data from breaches. Implementing transparent data usage policies can build trust with consumers.
2. Skill Shortages in Data Science
There is a significant skills gap in the current workforce concerning data science and machine learning expertise. Businesses should invest in training existing employees and consider partnerships with educational institutions to develop talent pools tailored to their needs.
3. Integration with Legacy Systems
Many businesses operate on legacy systems that may not easily integrate with new machine learning technologies. Undertaking a phased approach to modernization can facilitate smoother transitions and better utilization of new capabilities.
Looking Ahead: The Future of Machine Learning
The future of machine learning is bright, with promising developments on the horizon. Here are a few anticipated trends and innovations:
1. Explainable AI (XAI)
As more businesses adopt machine learning, the demand for transparency will grow. Explainable AI focuses on making machine learning decisions understandable to non-experts. This is crucial in fields such as healthcare and finance, where accountability is essential.
2. Edge Computing and Machine Learning
Edge computing is set to reshape how machine learning is deployed. By processing data closer to the source, businesses can reduce latency and bandwidth usage. This trend is particularly relevant for IoT devices that require real-time analytics.
3. Greater Collaboration Between Humans and AI
The synergy between human intelligence and machine learning will continue to enhance productivity. AI will serve as an augmenting tool that can assist professionals in making better decisions, fostering a collaborative environment.
Conclusion
In conclusion, the latest updates in machine learning reveal a dynamic landscape filled with opportunities for businesses willing to embrace change. By leveraging these advancements, organizations can enhance decision-making, improve operational efficiency, and deliver exceptional customer experiences. As we move through 2023 and beyond, staying informed on the trends and innovations in machine learning will be crucial for maintaining a competitive edge in the ever-evolving marketplace.
Resources for Further Learning
For those seeking to deepen their understanding of machine learning and its latest updates, consider the following resources:
- Machine Learning Consulting - Expert insights and consulting services for businesses.
- Kaggle - A platform for data science competitions and community engagement.
- Towards Data Science - A Medium publication featuring articles and tutorials on data science and machine learning.